Source: Hack a Day
Article note: HAD is the only appropriately skeptical place I saw this pop up. Everyone else went with "COLOR PHOTOS IN COMPLETE DARKNESS VIA AI", every word of which is a lie. They took pictures of inkjet printed pictures. Under three colors of controlled very near IR (if I showed you a 718nm LED you'd call it red) illumination. Then they characterized that particular printer's CMYK inks illuminated under the three frequencies of near-IR light, and used an overgrown parameter fitting tool to generate a linear system to translate the IR image to the visible light image (basically by distinguishing pigments and drawing the appropriate visible colors where they were printed). It would only take a couple hours to find and hand tune the constants from swatches, and you'd probably get better results.
It really is an illustration of everything wrong with academia.
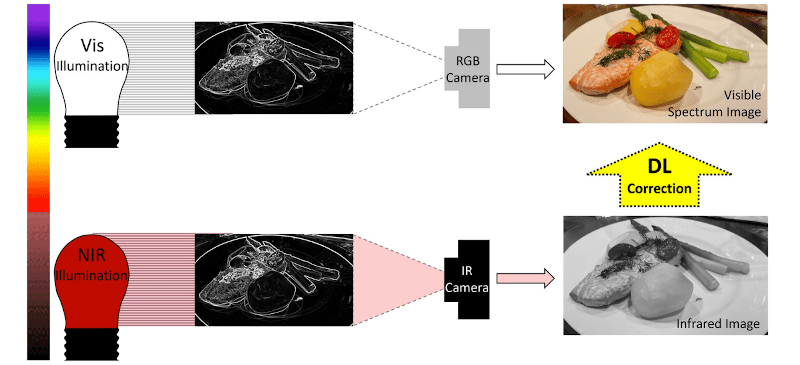
We’ve all gotten used to seeing movies depict people using night vision gear where everything appears as a shade of green. In reality the infrared image is monochrome, but since the human eye is very sensitive to green, the false-color is used to help the wearer distinguish the faintest glow possible. Now researchers from the University of California, Irvine have adapted night vision with artificial intelligence to produce correctly colored images in the dark. However, there is a catch, as the method might not be as general-purpose as you’d like.
Under normal illumination, white light has many colors mixed together. When light strikes something, it absorbs some colors and reflects others. So a pure red object reflects red and absorbs other colors. While some systems work by amplifying small amounts of light, those don’t work in total darkness. For that you need night vision gear that illuminates the scene with infrared light. Scientists reasoned that different objects might also absorb different kinds of infrared light. Training a system on what colors correspond to what absorption characteristics allows the computer to reconstruct the color of an image.
The only thing we found odd is that the training was on printed pictures of faces using a four-color ink process. So it seems like pointing the same camera in a dark room would give unpredictable results. That is, unless you had a huge database of absorption profiles. There’s a good chance, too, that there is overlap. For example, yellow paint from one company might look similar to blue paint from another company in IR, while the first company’s blue looks like something else. It is hard to imagine how you could compensate for things like that.
Still, it is an interesting idea and maybe it will lead to some other interesting night vision improvements. There could be a few niche applications, too, where you can train the system for the expected environment and the paper mentions a few of these.
Of course, if you have starlight, you can just use a very sensitive camera, but you still probably won’t get color. You can also build your own night vision gear without too much trouble.